撰文:周運(yùn)來,一個讀序列天書的公子哥,穩(wěn)健,瀟灑,大方,靠譜。大型測序工廠的螺絲釘,統(tǒng)計(jì)草原上的游牧者。 (https://www.jianshu.com/u/06ae70ef31bc) 編輯:陳同 --- 大師,大師,我想學(xué)習(xí)單細(xì)胞 ··· 閉上眼睛跟我來
單細(xì)胞測序有著漫長的過去,卻只有短暫的歷史—-誰說的! 
說她漫長是因?yàn)榈饺缃褚灿惺畮啄甑臍v史了(這里面兩個重要任務(wù):湯富酬老師2009年的文章使得單細(xì)胞測序成為現(xiàn)實(shí),郭國冀老師2010年的文章揭示對500多細(xì)胞進(jìn)行48個基因的單細(xì)胞RT-qPCR就可以進(jìn)行細(xì)胞類型區(qū)分,使得單細(xì)胞測序方向轉(zhuǎn)向大量細(xì)胞低深度測序),說她短暫是因?yàn)獒槍渭?xì)胞的分析工具越來越有意義開發(fā)周期卻越來越短。一般生物信息流程主要由軟件(安裝與參數(shù))、數(shù)據(jù)庫(結(jié)構(gòu)和生物學(xué)意義)和數(shù)據(jù)分析(統(tǒng)計(jì)學(xué)和編程)組成,目前單細(xì)胞分析用到的軟件主要是FastQC、Cellranger和R包Seurat、monocle;數(shù)據(jù)庫有相應(yīng)物種的參考基因組、KEGG、GO;數(shù)據(jù)分析部分主要基于count矩陣和差異表達(dá)數(shù)據(jù)用R或者Python來做。 cellranger 是10X genomics 公司為單細(xì)胞RNA測序分析量身打造的數(shù)據(jù)分析軟件,可以直接輸入Illumina 原始數(shù)據(jù)(raw base call ,BCL 或FASTQ)直接進(jìn)行文庫拆分、細(xì)胞拆分、輸出表達(dá)定量矩陣、降維(pca),聚類(Graph-based& K-Means)以及可視化(t-SNE)結(jié)果,結(jié)合配套的Loupe Cell Browser 給予研究者更多探索單細(xì)胞數(shù)據(jù)的機(jī)會。cellranger的高度集成化,使得單細(xì)胞測序數(shù)據(jù)探索變得更加簡單,研究者有更多的時(shí)間來做生物學(xué)意義的挖掘。
今天小編就要給大家介紹下這個可能成為行業(yè)標(biāo)準(zhǔn)的數(shù)據(jù)分析軟件——cellranger 。在這之前,還是先來了解一下10X genomics 單細(xì)胞測序的一般原理吧。 10X genomics
一個油滴 (GEM )=一個單細(xì)胞+一個凝膠微珠=一個scRNA-Seq,可以說這就是10X的基本技術(shù)原理。 
V2試劑盒產(chǎn)生的文庫結(jié)構(gòu): 
V3試劑盒產(chǎn)生的文庫結(jié)構(gòu): 
reads 1 :主要用來標(biāo)記(barcode、UMI以及reads的來源) reads 2 :與基因組比對 (配合UMI進(jìn)行定量) Barcode: 標(biāo)記細(xì)胞 UMI (Unique Molecular Identifier):標(biāo)記轉(zhuǎn)錄本 PolyT :捕獲成熟的RNA
10X genomics單細(xì)胞測序通過Barcode來標(biāo)記細(xì)胞和細(xì)胞計(jì)數(shù),UMI 來標(biāo)記轉(zhuǎn)錄本,這樣與參考基因組比對后就可以定量基因的表達(dá)量 (轉(zhuǎn)錄本數(shù)量,近乎絕對定量)。 在2019年3月7日10x官方網(wǎng)站對“單細(xì)胞基因表達(dá)入門”的直播視頻中提到(只是一場直播提到的信息,僅供參考): 由于10x單細(xì)胞測序的重復(fù)性較高,因此如無特殊原因,做生物學(xué)重復(fù)的意義不大; 如果細(xì)胞大小不一致,但直徑符合上機(jī)要求,對捕獲效率沒有明顯影響; 細(xì)胞重懸清洗后要保證鈣、鎂離子濃度較低,從而不影響反轉(zhuǎn)錄; 非常規(guī)形態(tài)或直徑較大的細(xì)胞可以采用抽核的方法進(jìn)行檢測; 當(dāng)被問及測序時(shí)需不需要加入標(biāo)準(zhǔn)物(如ERCC)的時(shí)候,官方給出的建議是不建議加ERCC(考慮到成本和影響細(xì)胞和基因的計(jì)數(shù))。
cell ranger pipelinecellranger單細(xì)胞分析流程主要分為:數(shù)據(jù)拆分 cellranger mkfastq、細(xì)胞定量 cellranger count、GEM整合 cellranger aggr、定制調(diào)整 cellranger reanalyze。還有一些用戶可能會用到的功能:mat2csv、mkgtf、mkref (構(gòu)建索引)、vdj、mkvdjref、testrun (測試軟件是否安裝成功和輸出結(jié)果的結(jié)構(gòu))、upload、sitecheck。 本文主要介紹常用的mkfastq , count , aggr 以及reanlyze 。 文庫拆分 cellranger mkfastq封裝了Illumina’s bcl2fastq軟件,用來拆分Illumina 原始數(shù)據(jù)(raw base call (BCL)),輸出 FASTQ 文件。 cellranger-cs/3.0.0 -h # 獲取幫助信息,篇幅有限就不展示了,可閱讀原文或自己運(yùn)行閱讀 # 不展示不代表不重要,做分析不讀參數(shù)解釋就是耍流氓
有以下兩種使用方式 $ cellranger mkfastq --id=tiny-bcl \ --run=/path/to/tiny_bcl \ --csv=cellranger-tiny-bcl-simple-1.2.0.csv
cellranger mkfastq Copyright (c) 2017 10x Genomics, Inc. All rights reserved. -------------------------------------------------------------------------------
Martian Runtime - 3.0.2-v3.2.0 Running preflight checks (please wait)... 2019-03-02 16:33:54 [runtime] (ready) ID.tiny-bcl.MAKE_FASTQS_CS.MAKE_FASTQS.PREPARE_SAMPLESHEET 2019-03-02 16:33:57 [runtime] (split_complete) ID.tiny-bcl.MAKE_FASTQS_CS.MAKE_FASTQS.PREPARE_SAMPLESHEET 2019-03-02 16:33:57 [runtime] (run:local) ID.tiny-bcl.MAKE_FASTQS_CS.MAKE_FASTQS.PREPARE_SAMPLESHEET.fork0.chnk0.main 2019-03-02 16:34:00 [runtime] (chunks_complete) ID.tiny-bcl.MAKE_FASTQS_CS.MAKE_FASTQS.PREPARE_SAMPLESHEET
cellranger-tiny-bcl-simple-1.2.0.csv 文件結(jié)構(gòu)
只有3列,第一列指定lane ID , 第二列指定樣本名稱 (來源于哪個GEM well,一般是一份生物樣品,一個GEM well對應(yīng)一個library,可以理解為下圖芯片中的一個channel),第三列指定index 的名稱,10X genomics的每個index代表4條具體的oligo序列,主要用于平衡文庫。拆分后的FASTQ文件是一個GEM well 中所有細(xì)胞的測序結(jié)果。 


$ cellranger mkfastq --id=tiny-bcl \ --run=/path/to/tiny_bcl \ --samplesheet=cellranger-tiny-bcl-samplesheet-1.2.0.csv
cellranger mkfastq Copyright (c) 2017 10x Genomics, Inc. All rights reserved. -------------------------------------------------------------------------------
Martian Runtime - 3.0.2-v3.2.0 Running preflight checks (please wait)... 2019-03-02 16:25:49 [runtime] (ready) ID.tiny-bcl.MAKE_FASTQS_CS.MAKE_FASTQS.PREPARE_SAMPLESHEET 2019-03-02 16:25:52 [runtime] (split_complete) ID.tiny-bcl.MAKE_FASTQS_CS.MAKE_FASTQS.PREPARE_SAMPLESHEET 2019-03-02 16:25:52 [runtime] (run:local) ID.tiny-bcl.MAKE_FASTQS_CS.MAKE_FASTQS.PREPARE_SAMPLESHEET.fork0.chnk0.main 2019-03-02 16:25:58 [runtime] (chunks_complete) ID.tiny-bcl.MAKE_FASTQS_CS.MAKE_FASTQS.PREPARE_SAMPLESHEET
如果使用samplesheet 文件(見下圖,一般不用),需要準(zhǔn)備的信息就多一些了,比如調(diào)整Reads 中的序列長度,而使用簡化版的csv 文件,cell ranger 可以識別所用試劑盒版本,然后自動化的調(diào)整reads 長度。 
拆分好之后的目錄結(jié)構(gòu)如下所示 ├── fastq_path │ ├── H35KCBCXY │ │ └── test_sample │ │ ├── test_sample_S1_L001_I1_001.fastq.gz #index序列 │ │ ├── test_sample_S1_L001_R1_001.fastq.gz #barcode umi │ │ └── test_sample_S1_L001_R2_001.fastq.gz #reads
注意下FASTQ文件的命名規(guī)律,如果我們直接拿到fastq文件,只要命名格式符合,也可以直接進(jìn)行后續(xù)分析。(圖中sample name,sample order類比一library name, library well number,見下面aggr部分) 
拆庫時(shí)可以加--qc 參數(shù)輸出序列質(zhì)量情況,保存在outs/qc_summary.json 中 (whitelist見aggr部分):
'sample_qc': { 'Sample1': { '5': { 'barcode_exact_match_ratio': 0.9336158258904611, 'barcode_q30_base_ratio': 0.9611993091728814, 'bc_on_whitelist': 0.9447542078230667, 'mean_barcode_qscore': 37.770630795934, 'number_reads': 2748155, 'read1_q30_base_ratio': 0.8947676653366835, 'read2_q30_base_ratio': 0.7771883245304577 }, 'all': { 'barcode_exact_match_ratio': 0.9336158258904611, 'barcode_q30_base_ratio': 0.9611993091728814, 'bc_on_whitelist': 0.9447542078230667, 'mean_barcode_qscore': 37.770630795934, 'number_reads': 2748155, 'read1_q30_base_ratio': 0.8947676653366835, 'read2_q30_base_ratio': 0.7771883245304577 } } }
cellranger countcount 是cellranger 最主要也是最重要的功能:完成細(xì)胞和基因的定量,也就是產(chǎn)生了我們用來做各種分析的基因表達(dá)矩陣。
cellranger count -h cellranger count (3.0.0) Copyright (c) 2018 10x Genomics, Inc. All rights reserved. -------------------------------------------------------------------------------
'cellranger count' quantifies single-cell gene expression.
The commands below should be preceded by 'cellranger':
Usage: count --id=ID [--fastqs=PATH] [--sample=PREFIX] --transcriptome=DIR [options] count <run_id> <mro> [options] count -h | --help | --version
Arguments: id A unique run id, used to name output folder [a-zA-Z0-9_-]+. fastqs Path of folder created by mkfastq or bcl2fastq. sample Prefix of the filenames of FASTQs to select. transcriptome Path of folder containing 10x-compatible reference.
Options: # Single Cell Gene Expression --description=TEXT Sample description to embed in output files. --libraries=CSV CSV file declaring input library data sources. --expect-cells=NUM Expected number of recovered cells. --force-cells=NUM Force pipeline to use this number of cells, bypassing the cell detection algorithm. --feature-ref=CSV Feature reference CSV file, declaring feature-barcode constructs and associated barcodes. --nosecondary Disable secondary analysis, e.g. clustering. Optional. --r1-length=NUM Hard trim the input Read 1 to this length before analysis. --r2-length=NUM Hard trim the input Read 2 to this length before analysis. --chemistry=CHEM Assay configuration. NOTE: by default the assay configuration is detected automatically, which is the recommened mode. You usually will not need to specify a chemistry. Options are: 'auto' for autodetection, 'threeprime' for Single Cell 3', 'fiveprime' for Single Cell 5', 'SC3Pv1' or 'SC3Pv2' or 'SC3Pv3' for Single Cell 3' v1/v2/v3, 'SC5P-PE' or 'SC5P-R2' for Single Cell 5'. paired-end/R2-only. Default: auto. --no-libraries Proceed with processing using a --feature-ref but no feature-barcoding data specified with the 'libraries' flag. --lanes=NUMS Comma-separated lane numbers. --indices=INDICES Comma-separated sample index set 'SI-001' or sequences. --project=TEXT Name of the project folder within a mkfastq or bcl2fastq-generated folder to pick FASTQs from.
# Martian Runtime --jobmode=MODE Job manager to use. Valid options: local (default), sge, lsf, or a .template file --localcores=NUM Set max cores the pipeline may request at one time. Only applies when --jobmode=local. --localmem=NUM Set max GB the pipeline may request at one time. Only applies when --jobmode=local. --mempercore=NUM Set max GB each job may use at one time. Only applies in cluster jobmodes. --maxjobs=NUM Set max jobs submitted to cluster at one time. Only applies in cluster jobmodes. --jobinterval=NUM Set delay between submitting jobs to cluster, in ms. Only applies in cluster jobmodes. --overrides=PATH The path to a JSON file that specifies stage-level overrides for cores and memory. Finer-grained than --localcores, --mempercore and --localmem. Consult the 10x support website for an example override file. --uiport=PORT Serve web UI at http://localhost:PORT --disable-ui Do not serve the UI. --noexit Keep web UI running after pipestance completes or fails. --nopreflight Skip preflight checks.
-h --help Show this message. --version Show version.
Note: 'cellranger count' can be called in two ways, depending on how you demultiplexed your BCL data into FASTQ files.
1. If you demultiplexed with 'cellranger mkfastq' or directly with Illumina bcl2fastq, then set --fastqs to the project folder containing FASTQ files. In addition, set --sample to the name prefixed to the FASTQ files comprising your sample. For example, if your FASTQs are named: subject1_S1_L001_R1_001.fastq.gz then set --sample=subject1
2. If you demultiplexed with 'cellranger demux', then set --fastqs to a demux output folder containing FASTQ files. Use the --lanes and --indices options to specify which FASTQ reads comprise your sample. This method is deprecated. Please use 'cellranger mkfastq' going forward.
—nosecondary 指定后不進(jìn)行后續(xù)的降維、聚類和可視化分析。一般是只獲得矩陣,后續(xù)分析用Seurat 。
輸出文件解釋 .outs ├── analysis【數(shù)據(jù)分析文件夾】 │ ├── clustering【聚類,圖聚類和k-means聚類】 │ ├── diffexp【差異分析】 │ ├── pca【主成分分析線性降維】 │ └── tsne【非線性降維信息】 ├── cloupe.cloupe【Loupe Cell Browser 輸入文件】 ├── filtered_feature_bc_matrix【很重要,Seurat, Monocle的輸入文件】 │ ├── barcodes.tsv.gz │ ├── features.tsv.gz │ └── matrix.mtx.gz ├── filtered_feature_bc_matrix.h5【過濾掉的barcode信息HDF5 format】 ├── metrics_summary.csv【CSV format數(shù)據(jù)摘要】 ├── molecule_info.h5【aggregate的時(shí)候會用到的文件】 ├── raw_feature_bc_matrix【原始barcode信息】 │ ├── barcodes.tsv.gz │ ├── features.tsv.gz │ └── matrix.mtx.gz ├── possorted_genome_bam.bam【比對文件】 ├── possorted_genome_bam.bam.bai【索引文件】 ├── raw_feature_bc_matrix.h5【原始barcode信息HDF5 format】 ├── web_summary.html【網(wǎng)頁簡版報(bào)告以及可視化】 └── *_gene_bar.csv_temp【過程文件】
GEM文庫整合 cellranger aggr當(dāng)實(shí)驗(yàn)中用到了多個GEM well ,并且想放在一起分析時(shí),需要先用cellranger count 分析各個來自于一個GEM well 的fastq文件 (與是否同一個lane 測序沒關(guān)系),然后再用cellranger aggr 進(jìn)行整合。 $ cd /home/jdoe/runs $ cellranger aggr --id=AGG123 \ --csv=AGG123_libraries.csv \ --normalize=mapped
library_id,molecule_h5 LV123,/opt/runs/LV123/outs/molecule_info.h5 LB456,/opt/runs/LB456/outs/molecule_info.h5 LP789,/opt/runs/LP789/outs/molecule_info.h5
library_id,molecule_h5,batch LV123,/opt/runs/LV123/outs/molecule_info.h5,v2_lib LB456,/opt/runs/LB456/outs/molecule_info.h5,v3_lib LP789,/opt/runs/LP789/outs/molecule_info.h5,v3_lib
什么是 GEM Wells 每個GEM well 是10X芯片上的一個單獨(dú)的區(qū)室,從barcode 池 (barcode whitelist ,前面--qc 的結(jié)果中有,評估barcode 測序準(zhǔn)確度,進(jìn)而影響細(xì)胞鑒定準(zhǔn)確度)中隨機(jī)獲取barcode 用于標(biāo)記細(xì)胞。為了保證整合多個文庫時(shí)barcode 不發(fā)生沖突,通常會在barcode 后面加一個數(shù)字,標(biāo)記其來源的GEM well ,如AGACCATTGAGACTTA-1 和AGACCATTGAGACTTA-2 ,barcode 序列一樣,但來源于不同的GEM well ,也是不同的細(xì)胞。 Outputs: - Aggregation metrics summary HTML: /home/jdoe/runs/AGG123/outs/web_summary.html - Aggregation metrics summary JSON: /home/jdoe/runs/AGG123/outs/summary.json - Secondary analysis output CSV: /home/jdoe/runs/AGG123/outs/analysis - Filtered feature-barcode matrices MEX: /home/jdoe/runs/AGG123/outs/filtered_feature_bc_matrix - Filtered feature-barcode matrices HDF5: /home/jdoe/runs/AGG123/outs/filtered_feature_bc_matrix.h5 - Unfiltered feature-barcode matrices MEX: /home/jdoe/runs/AGG123/outs/raw_feature_bc_matrix - Unfiltered feature-barcode matrices HDF5: /home/jdoe/runs/AGG123/outs/raw_feature_bc_matrix.h5 - Unfiltered molecule-level info: /home/jdoe/runs/AGG123/outs/raw_molecules.h5 - Barcodes of cell-containing partitions: /home/jdoe/runs/AGG123/outs/cell_barcodes.csv - Copy of the input aggregation CSV: /home/jdoe/runs/AGG123/outs/aggregation.csv - Loupe Cell Browser file: /home/jdoe/runs/AGG123/outs/cloupe.cloupe
定制調(diào)整 cellranger reanalyze相比于count 和aggr ,reanalyze 接受更多的可選的參數(shù),可以說count 和aggr 中的后續(xù)分析只是屬于探索性分析,這里的reanalyze 才是真正開始接觸故事的真相。 $ cellranger reanalyze -h cellranger reanalyze (3.0.0) Copyright (c) 2018 10x Genomics, Inc. All rights reserved. -------------------------------------------------------------------------------
'cellranger reanalyze' performs secondary analysis (dimensionality reduction, clustering and differential expression) on a feature-barcode matrix generated by the 'cellranger count' or 'cellranger aggr' pipelines.
The analysis takes parameter settings from a CSV file. Please see the following URL for details on the CSV format: support.10xgenomics.com/single-cell/software
This pipeline does not support multi-genome samples.
The commands below should be preceded by 'cellranger':
Usage: reanalyze --id=ID --matrix=MATRIX_H5 [options] reanalyze <run_id> <mro> [options] reanalyze -h | --help | --version
Arguments: id A unique run id, used to name output folder [a-zA-Z0-9_-]+. matrix A feature-barcode matrix containing data for one genome. Should be the filtered version, unless using --force-cells.
Options: # Analysis --description=TEXT Sample description to embed in output files. --params=PARAMS_CSV A CSV file specifying analysis parameters. Optional. --barcodes=BARCODE_CSV A CSV file containing a list of cell barcodes to use for reanalysis, e.g. barcodes exported from Loupe Cell Browser. Optional. --genes=GENES_CSV A CSV file containing a list of feature IDs to use for reanalysis. For gene expression, this should correspond to the gene_id field in the reference GTF should be (e.g. ENSG... for ENSEMBL-based references). Optional. --exclude-genes=GENES_CSV A CSV file containing a list of feature IDs to exclude for reanalysis. For gene expression, this should correspond to the gene_id field in the reference GTF (e.g., ENSG... for ENSEMBL-based references). The exclusion is applied after --genes. Optional. --agg=AGGREGATION_CSV If the input matrix was produced by 'cellranger aggr', you may pass the same aggregation CSV in order to retain per-library tag information in the resulting .cloupe file. This argument is required to enable chemistry batch correction Optional. --force-cells=NUM Force pipeline to use this number of cells, bypassing the cell detection algorithm. Optional.
# Martian Runtime --jobmode=MODE Job manager to use. Valid options: local (default), sge, lsf, or a .template file --localcores=NUM Set max cores the pipeline may request at one time. Only applies when --jobmode=local. --localmem=NUM Set max GB the pipeline may request at one time. Only applies when --jobmode=local. --mempercore=NUM Set max GB each job may use at one time. Only applies in cluster jobmodes. --maxjobs=NUM Set max jobs submitted to cluster at one time. Only applies in cluster jobmodes. --jobinterval=NUM Set delay between submitting jobs to cluster, in ms. Only applies in cluster jobmodes. --overrides=PATH The path to a JSON file that specifies stage-level overrides for cores and memory. Finer-grained than --localcores, --mempercore and --localmem. Consult the 10x support website for an example override file. --uiport=PORT Serve web UI at http://localhost:PORT --disable-ui Do not serve the UI. --noexit Keep web UI running after pipestance completes or fails. --nopreflight Skip preflight checks.
-h --help Show this message. --version Show version.
Note: 'cellranger reanalyze' can be called in two ways, depending on how you demultiplexed your BCL data into FASTQ files.
1. If you demultiplexed with 'cellranger mkfastq' or directly with Illumina bcl2fastq, then set --fastqs to the project folder containing FASTQ files. In addition, set --sample to the name prefixed to the FASTQ files comprising your sample. For example, if your FASTQs are named: subject1_S1_L001_R1_001.fastq.gz then set --sample=subject1
2. If you demultiplexed with 'cellranger demux', then set --fastqs to a demux output folder containing FASTQ files. Use the --lanes and --indices options to specify which FASTQ reads comprise your sample. This method is deprecated. Please use 'cellranger mkfastq' going forward.
$ cd /home/jdoe/runs $ ls -1 AGG123/outs/*.h5 # verify the input file exists AGG123/outs/filtered_feature_bc_matrix.h5 AGG123/outs/filtered_molecules.h5 AGG123/outs/raw_feature_bc_matrix.h5 AGG123/outs/raw_molecules.h5 $ cellranger reanalyze --id=AGG123_reanalysis \ --matrix=AGG123/outs/filtered_feature_bc_matrix.h5 \ --params=AGG123_reanalysis.csv
Outputs: - Secondary analysis output CSV: /home/jdoe/runs/AGG123_reanalysis/outs/analysis_csv - Secondary analysis web summary: /home/jdoe/runs/AGG123_reanalysis/outs/web_summary.html - Copy of the input parameter CSV: /home/jdoe/runs/AGG123_reanalysis/outs/params_csv.csv - Copy of the input aggregation CSV: /home/jdoe/runs/AGG123_reanalysis/outs/aggregation_csv.csv - Loupe Cell Browser file: /home/jdoe/runs/AGG123_reanalysis/outs/cloupe.cloupe
數(shù)據(jù)分析概述Cell Ranger是由10x genomic公司官方提供的專門用于其單細(xì)胞轉(zhuǎn)錄組數(shù)據(jù)分析的軟件包。Cell Ranger將前面產(chǎn)生的fastq 測序數(shù)據(jù)比對到參考基因組上,然后進(jìn)行基因表達(dá)定量,生成細(xì)胞-基因表達(dá)矩陣,并基于此進(jìn)行細(xì)胞聚類和差異表達(dá)分析。 比對基因組比對 Cell Ranger使用star比對軟件將reads比對到參考基因組上后使用GTF注釋文件進(jìn)行校正,并區(qū)分出外顯子區(qū)、內(nèi)含子區(qū)、基因間區(qū)。 具體的區(qū)分規(guī)則(mapping QC)為:至少50% 比對到外顯子上reads記為外顯子區(qū)、將比對到非外顯子區(qū)且與內(nèi)含子區(qū)有交集的reads記為內(nèi)含子區(qū),除此之外均為基因間區(qū)。 MAPQ 校正 對于比對到單個外顯子位點(diǎn)但同時(shí)比對到1個或多個非外顯子位點(diǎn)的reads,對外顯子位點(diǎn)進(jìn)行優(yōu)先排序,并將reads記為帶有MAPQ 255的外顯子位點(diǎn)。 轉(zhuǎn)錄組比對 Cell Ranger進(jìn)一步將外顯子reads與參考轉(zhuǎn)錄本比對,尋找兼容性。注釋到單個基因信息的reads認(rèn)為是一個特異的轉(zhuǎn)錄本,只有注釋到轉(zhuǎn)錄本的reads才用于UMI計(jì)數(shù)。
參考基因組目錄結(jié)構(gòu) (mkref 生成,也可直接從官網(wǎng)下載): ├── fasta │ └── genome.fa ├── genes │ └── genes.gtf ├── pickle │ └── genes.pickle ├── README.BEFORE.MODIFYING ├── reference.json ├── star │ ├── chrLength.txt │ ├── chrNameLength.txt │ ├── chrName.txt │ ├── chrStart.txt │ ├── exonGeTrInfo.tab │ ├── exonInfo.tab │ ├── geneInfo.tab │ ├── Genome │ ├── genomeParameters.txt │ ├── SA │ ├── SAindex │ ├── sjdbInfo.txt │ ├── sjdbList.fromGTF.out.tab │ ├── sjdbList.out.tab │ └── transcriptInfo.tab └── version
細(xì)胞計(jì)數(shù)(cell QC)液滴型scRNA-seq方法中只有一小部分的液滴包含珠狀物和一個完整細(xì)胞。然而生物實(shí)驗(yàn)不會那么理想,有些RNA會從死細(xì)胞或破損細(xì)胞中漏出來。所以沒有完整細(xì)胞的液滴有可能捕獲周圍環(huán)境游離出的少了RNA并且走完測序環(huán)節(jié)出現(xiàn)在最終測序結(jié)果中。液滴大小、擴(kuò)增效率和測序環(huán)節(jié)中的波動會導(dǎo)致”背景”和真實(shí)細(xì)胞最終獲得的文庫大小變化很大,使得區(qū)分哪些文庫來源于背景哪些來源于真實(shí)細(xì)胞變得復(fù)雜。具體見Hemberg-lab單細(xì)胞轉(zhuǎn)錄組數(shù)據(jù)分析(四)。 Cell Ranger 3.0引入了一種改進(jìn)的細(xì)胞計(jì)數(shù)算法,該算法能夠更好地識別低RNA含量的細(xì)胞群體,特別是當(dāng)?shù)蚏NA含量的細(xì)胞與高RNA含量的細(xì)胞混合時(shí)。該算法分為兩步: 在第一步中,使用之前的Cell Ranger細(xì)胞計(jì)數(shù)算法識別高RNA含量細(xì)胞的主要模式,使用基于每個barcode的UMI總數(shù)的cutoff值。Cell Ranger將期望捕獲的細(xì)胞數(shù)量N作為輸入(see —expect-cells)。然后將barcodes按照各自的UMI總數(shù)由高到低進(jìn)行排序,取前N個UMI數(shù)值的99%分位數(shù)為最大估算UMI總數(shù)(m),將UMI數(shù)目超過m/10的barcodes標(biāo)記的細(xì)胞視為真實(shí)細(xì)胞。 在第二步中,選擇一組具有低UMI計(jì)數(shù)的barcode,這些barcode可能表示“空的”GEM分區(qū),建立RNA圖譜背景模型。利用Simple Good-Turing smoothing平滑算法,對典型空GEM集合中未觀測到的基因進(jìn)行非零模型估計(jì)。最后,將第一步中未作為細(xì)胞計(jì)數(shù)的barcode RNA圖譜與背景模型進(jìn)行比較,其RNA譜與背景模型存在較大差異的barcode用于區(qū)分包含細(xì)胞的barcode和空barcode。
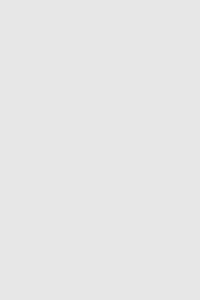
多態(tài)率估計(jì)(Estimating Multiplet Rates)當(dāng)有多個參考基因組(如人H和鼠M)時(shí),Cell Ranger可以通過多基因組分析區(qū)分多物種混合建庫的樣品,主要根據(jù)barcode內(nèi)每個物種對應(yīng)的UMI數(shù)量進(jìn)行區(qū)分,將其分成H和M兩類。最后還會根據(jù)H,M各自UMI的分布和最大似然估計(jì)法估計(jì)多細(xì)胞比例(multiplet rate ),即 (H,M)、(H,H)、(M,M)三種類型的多細(xì)胞占比。 基因表達(dá)分析(Secondary Analysis of Gene Expression)盡管我們的表達(dá)矩陣經(jīng)過重重QC,但是單細(xì)胞高通量的數(shù)據(jù)還是表現(xiàn)出高緯度、稀疏性、非正態(tài)分布的特點(diǎn),每一點(diǎn)都是對傳統(tǒng)數(shù)據(jù)分析方法的挑戰(zhàn)。于是越來越多的新方法被開發(fā)出來,主要借鑒多元分析和機(jī)器學(xué)習(xí)等傳統(tǒng)生物統(tǒng)計(jì)教材很少教授的方法。越來越多的機(jī)器學(xué)習(xí)方法應(yīng)用到高通量數(shù)據(jù)分析中來,那么我們就需要了解機(jī)器學(xué)習(xí)的三個要素; 公式(方法) = 模型 (目的) + 策略(評價(jià)) + 算法(實(shí)現(xiàn))
降維分析(Dimensionality Reduction)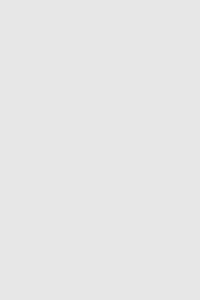
流形學(xué)習(xí)(manifold learning )是機(jī)器學(xué)習(xí)、模式識別中的一種方法,在維數(shù)約簡方面具有廣泛的應(yīng)用。它的主要思想是將高維的數(shù)據(jù)映射到低維,使該低維的數(shù)據(jù)能夠反映原高維數(shù)據(jù)的某些本質(zhì)結(jié)構(gòu)特征。流形學(xué)習(xí)的前提是有一種假設(shè),即某些高維數(shù)據(jù),實(shí)際是一種低維的流形結(jié)構(gòu)嵌入在高維空間中。流形學(xué)習(xí)的目的是將其映射回低維空間中,揭示其本質(zhì)。 針對單細(xì)胞測速數(shù)據(jù)特點(diǎn),一般為了提取基因表達(dá)矩陣最重要的特征采用降維分析將多維數(shù)據(jù)的差異投影在低維度上,進(jìn)而揭示復(fù)雜數(shù)據(jù)背后的規(guī)律。Cell Ranger先采用基于IRLBA算法的主成分分析(Principal Components Analysis,PCA))將數(shù)據(jù)集的維數(shù)從(Cell x genes)改變?yōu)?Cell x M,M是主成分?jǐn)?shù)量)。然后采用非線性降維算法t-SNE)將降維后的數(shù)據(jù)展示在2維或三維空間中,細(xì)胞之間的基因表達(dá)模式越相似,在t-SNE圖中的距離也越接近。 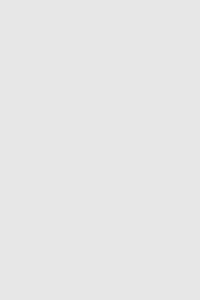
聚類分析(Clustering)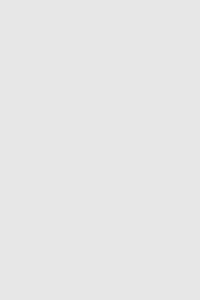
聚類是把相似的對象通過靜態(tài)分類的方法分成不同的組別或者更多的不連續(xù)子集(subset),這樣讓在同一個子集中的成員對象都有相似的一些屬性。在單細(xì)胞研究中,聚類分析是識別細(xì)胞異質(zhì)性(heterogeneity)常用的算法。 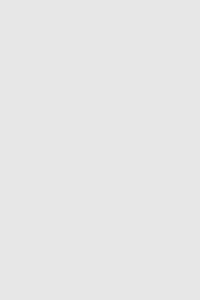
在PCA空間中,Cell Ranger分別采用Graph-based 和K-Means 兩種不同的聚類方法對細(xì)胞進(jìn)行聚類: 圖聚類算法包括兩步:首先用PCA降維的數(shù)據(jù)構(gòu)建一個細(xì)胞間的k近鄰稀疏矩陣,即將一個細(xì)胞與其歐式距離上最近的k個細(xì)胞聚為一類,然后在此基礎(chǔ)上用Louvain算法進(jìn)行模塊優(yōu)化 (Blondel, Guillaume, Lambiotte, & Lefebvre, 2008),旨在找到圖中高度連接的模塊。最后通過層次聚類將位于同一區(qū)域內(nèi)沒有差異表達(dá)基因(B-H adjusted p-value 低于0.05)的cluster進(jìn)一步融合,重復(fù)該過程直到?jīng)]有clusters可以合并。 K-Means聚類是無監(jiān)督的機(jī)器學(xué)習(xí)算法。在PCA降維的空間中隨機(jī)選取的k個初始質(zhì)心點(diǎn),將每個點(diǎn)劃分到最近的質(zhì)心,形成K個簇 ,然后對于每一個cluster重新計(jì)算質(zhì)心并再次進(jìn)行劃分,重復(fù)該過程直到收斂。與圖聚類算法的k意義不同,這里的k是事先給定的亞群數(shù)目。 差異分析(Differential Expression)為了找到不同細(xì)胞亞群之間的差異基因,Cell Ranger使用改進(jìn)的sSeq方法(基于負(fù)二項(xiàng)檢驗(yàn); Yu, Huber, & Vitek, 2013)。當(dāng)UMI counts數(shù)值較大時(shí),為加快分析速度,Cell Ranger會自動切換到edgeR進(jìn)行beta檢驗(yàn)(Robinson & Smyth, 2007)。通過樣品的一個亞群與該樣品的所有其他亞群進(jìn)行比較,得到該亞群細(xì)胞與其他亞群細(xì)胞之間的差異基因列表。 Cell Ranger’s implementation differs slightly from that in the paper: in the sSeq paper, the authors recommend using DESeq’s geometric mean-based definition of library size. Cell Ranger instead computes relative library size as the total UMI counts for each cell divided by the median UMI counts per cell. As with sSeq, normalization is implicit in that the per-cell library-size parameter is incorporated as a factor in the exact-test probability calculations. 化學(xué)批次校正(Chemistry Batch Correction)為了校正V2V3化學(xué)試劑之間的批次效應(yīng),Cell Ranger采用一種基于mutual nearest neighbors (MNN; (Haghverdi et al, 2018)的算法來識別批次之間的相似細(xì)胞亞群。MNN定義為來自彼此最近鄰集合中包含的兩個不同批次的細(xì)胞群。使用批次之間匹配的細(xì)胞亞群,將多個批次合并在一起(Hie et al, 2018)。MNN對中細(xì)胞間表達(dá)值的差異提供了批次效應(yīng)的估計(jì)。每個細(xì)胞校正向量的加權(quán)平均值用來估計(jì)批次效應(yīng)。 批次效應(yīng)得分(batch effect score )被定義為定量測量校正前后的批次效應(yīng)。對于每個細(xì)胞,計(jì)算其k個最近的細(xì)胞(nearest-neighbors)中有m個屬于同一批次,并在沒有批次效應(yīng)時(shí)將其標(biāo)準(zhǔn)化為相同批次細(xì)胞的期望值M 。批次效應(yīng)得分計(jì)算為隨機(jī)抽取10%的細(xì)胞總數(shù)S中的上述度量的平均值。如果沒有批次效應(yīng),我們可以預(yù)期每個單元格的最近鄰居將在所有批次中均勻共享,批次效應(yīng)得分接近1。 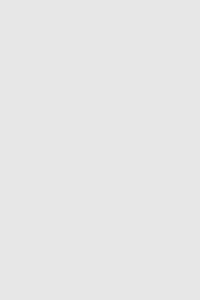
最近單細(xì)胞的小伙伴都在看的一篇文章,不知道你看了沒:單細(xì)胞測序(scRNA-seq)數(shù)據(jù)處理必知必會:
|